Transform Your Business with Spam Filtering Using Machine Learning
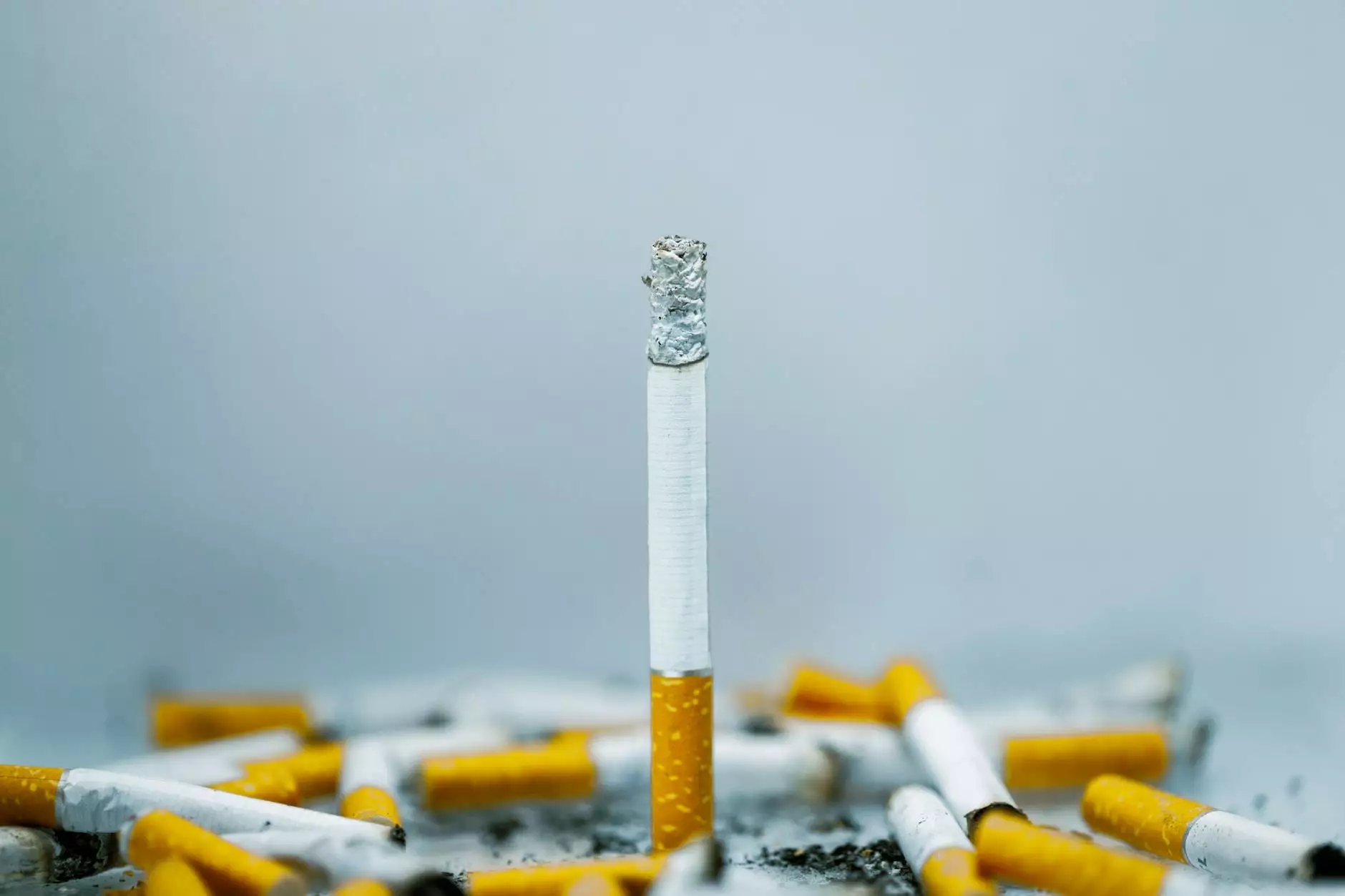
In today's digital landscape, effective communication is a cornerstone of successful business operations. However, the rise of unsolicited emails, or spam, poses significant challenges. These malicious or irrelevant messages not only clutter inboxes but can also lead to severe security breaches. To mitigate these threats, businesses are increasingly turning to advanced technologies, particularly spam filtering using machine learning, to bolster their defenses and streamline communication.
Understanding Spam and Its Implications
Spam refers to unsolicited emails sent in bulk, often for advertising or phishing purposes. The implications of spam on a business can be detrimental:
- Decreased Productivity: Employees spend unnecessary time sifting through spam emails instead of focusing on essential tasks.
- Security Risks: Spam can contain malicious links, leading to potential malware infections and data breaches.
- Damage to Reputation: Falling victim to phishing attacks can tarnish a company's reputation and erode customer trust.
What Is Machine Learning?
Machine learning (ML) is a subset of artificial intelligence that enables systems to learn from data and improve their accuracy over time without being explicitly programmed. In the context of spam filtering, ML algorithms analyze patterns and characteristics of emails to accurately classify them as either spam or legitimate messages.
How Spam Filtering Using Machine Learning Works
Spam filtering with machine learning involves several key steps:
1. Data Collection
ML models require vast amounts of data to learn effectively. For spam filtering, this entails collecting a diverse range of emails that are labeled as either spam or not spam.
2. Feature Extraction
In this phase, relevant features or attributes are extracted from the emails. Common features include:
- Sender's email address
- Email subject line
- Content of the email
- Frequency of specific keywords
3. Training the Model
Once the data is collected and features are extracted, the next step is to train the machine learning model. This involves feeding the model the labeled data so it can learn the distinguishing features of spam and non-spam emails.
4. Testing and Validation
After training the model, it's essential to evaluate its performance using a separate dataset. This helps ensure that the model accurately identifies spam without misclassifying legitimate emails.
5. Deployment
Once validated, the spam filtering model can be deployed in real-time to scan incoming emails and make decisions based on learned patterns.
Advantages of Utilizing Spam Filtering Using Machine Learning
Implementing spam filtering through machine learning offers numerous benefits for businesses:
Enhanced Accuracy
Machine learning models can adapt and improve over time as they are exposed to more data. This leads to significantly higher accuracy in identifying spam compared to traditional filtering methods.
Reduced False Positives
Advanced models minimize the occurrence of false positives—legitimate emails being incorrectly flagged as spam—ensuring that important messages are not lost.
Scalability
Machine learning solutions can scale seamlessly as the volume of emails increases, allowing businesses to handle growth without compromising efficiency.
Challenges in Spam Filtering Using Machine Learning
Despite its numerous advantages, spam filtering with machine learning is not without its challenges:
Data Privacy Concerns
Businesses must ensure that they handle sensitive data responsibly when collecting emails for training their models. Adhering to data protection regulations like GDPR is crucial.
Continuous Learning Requirement
The dynamic nature of spam tactics means that models must continuously learn and adapt to new types of spam. This requires ongoing monitoring and retraining of the models.
Best Practices for Implementing Spam Filtering Using Machine Learning
To achieve the most effective spam filtering results, consider the following best practices:
1. Use a Comprehensive Dataset
Ensure your training data includes a diverse array of spam and legitimate emails to allow the model to learn effectively.
2. Implement Regular Updates
Regularly update your model with new data, incorporating recent spam tactics and trends to enhance its learning capabilities.
3. Monitor Performance
Continuously monitor the performance of your spam filtering system. Adjust parameters and retrain models as needed to maintain accuracy.
Conclusion
In conclusion, spam filtering using machine learning represents a game-changing innovation for businesses looking to enhance their email security and operational efficiency. By reducing spam-related risks, improving communication, and ensuring the safety of sensitive information, companies can not only protect themselves but also enhance their overall productivity. As threats evolve, leveraging machine learning for spam filtering will undoubtedly become an essential component of any robust IT strategy.
For businesses ready to adopt cutting-edge security solutions, partnering with experts in the field of IT services, such as Spambrella, can provide the necessary guidance and technology to implement effective spam filtering systems customized to your needs.
Take control over your email communications today and transform your business’s spam filtering processes with machine learning technology!